Unlocking the Potential of Generative AI for Synthetic Data Generation
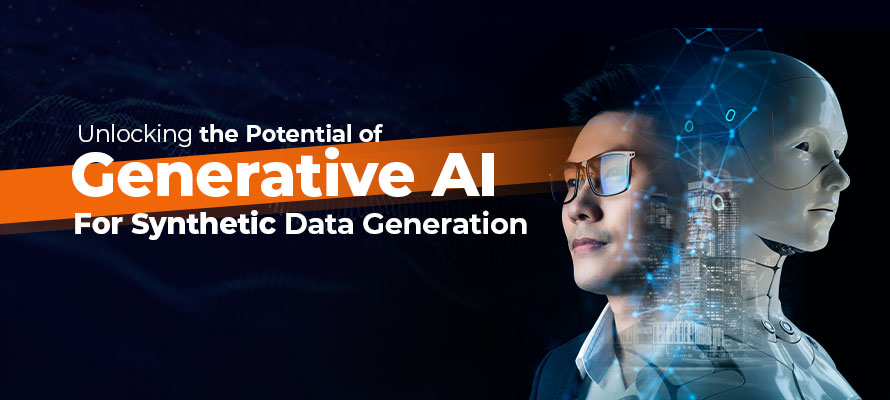
Written by Syed Usman Chishti
Subject Matter Expert
September 14, 2023
In today’s data-driven world, the ability to generate synthetic data has become a powerful tool with applications ranging from software development to machine learning. Generative AI, a subset of artificial intelligence, has emerged as the driving force behind creating new data that mimics the patterns and relationships present in real-world datasets.
This blog delves into generative AI, exploring its capabilities and potential in generating synthetic data for diverse domains like software development, data analytics, and machine learning.
Understanding Generative AI
Generative AI stands at the forefront of cutting-edge technologies, empowering machines to create new data that closely resembles existing datasets. These algorithms learn the intricate underlying patterns and relationships in the original data, harnessing this knowledge to craft novel data points that maintain consistency with the source dataset. This field is rapidly evolving, promising to revolutionize data generation and utilization.
The Role of Generative AI in Synthetic Data Generation
Generative AI’s capacity to produce synthetic data is immensely significant across various domains. It enables the creation of lifelike virtual environments that serve as excellent training and simulation grounds. Additionally, generative AI is pivotal in supplying new data for training machine learning models.
Here is a simpler breakdown:
- Privacy Preservation: Generative AI can create synthetic data that closely mimics real data’s statistical properties and patterns while not containing any personally identifiable information (PII). This is particularly important in healthcare, finance, and education industries, where data privacy regulations are stringent.
- Data Diversity: Synthetic data can be generated to represent a wide range of scenarios, outliers, and edge cases that might not be present in the limited real data available. This diversity can improve the robustness of machine learning models and help them generalize better.
- Data Imbalance: In cases where real data is imbalanced, with one class significantly outnumbering others, generative AI can balance the dataset by creating synthetic examples of the minority class. This can lead to more accurate and fairer machine learning models.
Forms of Synthetic Data
Synthetic data comes in various forms, each serving a specific purpose in data generation and analysis. The article will explore these forms, shedding light on how generative AI can create:
- Tabular Data: This form generates datasets mirroring real-world data in structure and statistical attributes, paving the way for applications in fields such as finance or customer behavior analysis
- Time Series Data: When real-world time series data is scarce or expensive, generative AI can craft datasets replicating these time-based sequences’ characteristics, opening doors to improved forecasting and modeling.
- Image and Video Data: Generative AI can fabricate realistic images and videos for machine learning models or simulations, enriching training datasets and enhancing model accuracy.
- Text Data: Generative AI facilitates tasks like natural language processing and creating diverse training data for machine learning models by generating coherent and realistic text.
- Sound Data: Generative AI contributes to refining sound-based machine learning applications and simulations by producing authentic sound data.
Patterns and Behaviors in Synthetic Data Generation
Here are some patterns and behaviors that can be easily modeled with synthetic data generation.
- Randomness: Purely random data can be generated using basic techniques like random number generators.
- Text Data: Techniques from natural language processing can be employed to generate text data.
- Time Series: Time series data, such as stock prices, can be generated using specialized models like ARIMA and LSTM.
- Proportions and Percentages: Data involving proportions can be generated using distributions like beta or Dirichlet.
Challenges in Creating Synthetic Data Generation
Creating synthetic data comes with various challenges, such as:
- Technical Difficulty: Accurately modeling complex real-world behaviors with synthetic data presents a formidable challenge.
- Bias Concerns: Synthetic data’s malleability makes it susceptible to producing biased results, emphasizing the need for cautious generation techniques.
- Privacy Safeguarding: While generating synthetic data, it’s crucial to ensure that sensitive information remains concealed.
- Data Model Quality: The accuracy of the data model directly impacts the validity of conclusions drawn from synthetic data.
- Time and Effort: Generating synthetic data demands significant time and effort.
Conclusion
Generative AI’s potential to generate synthetic data is a game-changer across industries. This article has offered a comprehensive exploration of the capabilities of generative AI and its role in producing synthetic data for diverse applications. From tabular to image data and challenges to solutions, the power of generative AI in reshaping data generation and utilization is undeniable.
Royal Cyber is a leading consultant for generative AI and can help you build a custom conversational AI solution. Feel free to get in touch with us for further discussion.